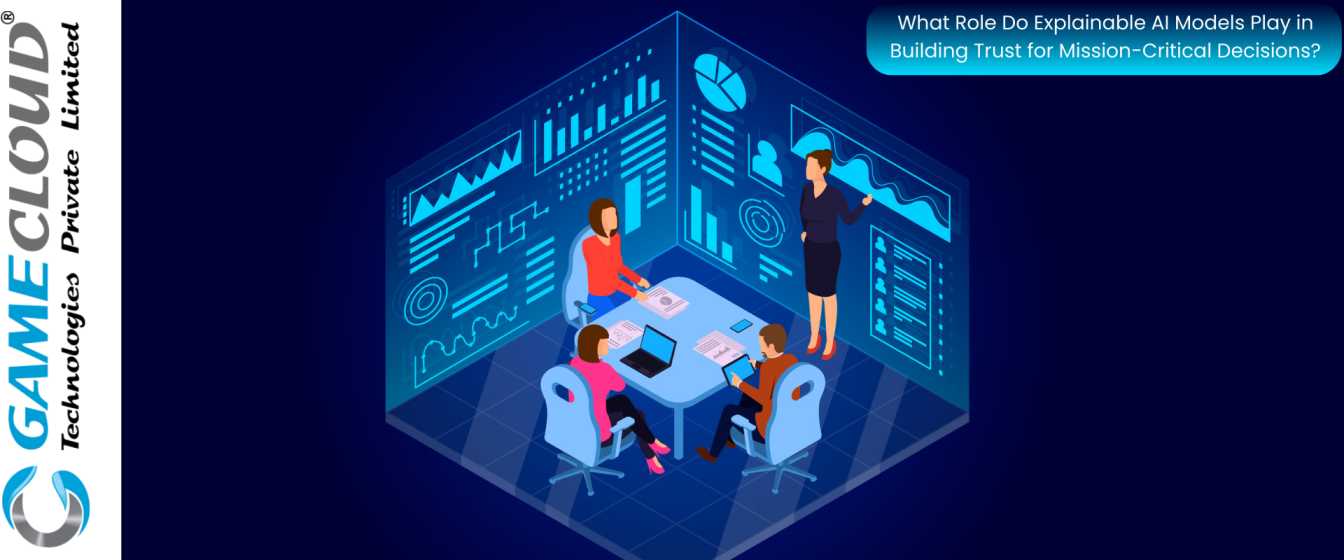
AI has become integral to various sectors, including healthcare, finance, defense, and transportation. In these mission-critical areas, AI systems often make decisions that can significantly impact human lives and societal outcomes. However, the opaque nature of many AI models, often referred to as “black boxes,” poses challenges in understanding and trusting their decisions. This is where Explainable AI comes into play, offering transparency and fostering trust in AI-driven decision-making processes.
Understanding Explainable AI
Explainable AI refers to methods and techniques that make the decision-making processes of AI systems transparent and understandable to humans. Unlike traditional AI models, which operate without providing insights into their reasoning, XAI aims to clarify how specific decisions are made, enabling users to comprehend and trust the outcomes.
For example, a typical black-box AI model might classify a patient as at high risk for a particular disease without offering any explanation. An explainable model, on the other hand, would outline the contributing factors, such as family history, lifestyle habits, or specific medical test results. By providing this level of detail, XAI empowers stakeholders—whether they are medical professionals, financial analysts, or defense personnel—to better understand and trust the system’s conclusions.
The Importance of Trust in Mission-Critical Decisions
In mission-critical domains, such as healthcare, finance, and defense, the stakes are exceptionally high. The decisions made by AI systems in these areas can have life-altering consequences, making trust a non-negotiable factor.
For instance, in healthcare, AI systems are increasingly used to assist in diagnosing diseases, identifying treatment options, and predicting patient outcomes. Imagine an AI model suggesting an aggressive treatment plan for a cancer patient but offering no explanation for its recommendation. Without transparency, medical professionals and patients may question the reliability of the decision, leading to hesitation or rejection of AI-guided care.
Similarly, in finance, AI is employed to assess creditworthiness, detect fraud, and optimize investments. A credit decision that denies a loan application without explaining the rationale can frustrate customers and raise concerns about bias or discrimination. Explainable AI can bridge this gap by clearly outlining the factors that influenced the decision, such as income, credit history, or debt-to-income ratio.
In defense, AI systems are used for surveillance, threat detection, and strategic decision-making. In these contexts, explainability becomes even more critical as decisions often involve national security or human lives. Transparency ensures that military or defense personnel can verify AI-driven recommendations and make informed judgments.
How Explainable AI Builds Trust
Explainable AI builds trust by providing stakeholders with the tools to understand and verify AI decisions. Transparency ensures that users can evaluate whether an AI model’s logic aligns with ethical standards, regulatory requirements, and human values.
- Ensuring Accountability: XAI enhances accountability by enabling users to trace the reasoning behind AI decisions. For example, if an AI-powered hiring tool is found to favor certain demographics, explainability can help identify and rectify biases in the algorithm.
- Facilitating Collaboration: In healthcare, explainable AI fosters collaboration between clinicians and AI systems. When doctors understand why an AI model suggests a particular diagnosis or treatment, they are more likely to integrate its recommendations into patient care.
- Improving Decision Confidence: Explainability increases confidence in AI decisions by providing assurance that the outcomes are based on logical and fair reasoning. This is especially important in high-stakes situations, such as disaster response or emergency management, where trust in AI can lead to faster, more effective decision-making.
- Regulatory Compliance: Many industries face strict regulations regarding transparency and fairness. For instance, financial institutions must ensure that their algorithms do not discriminate based on race, gender, or other protected characteristics. Explainable AI helps organizations meet these requirements by making decision-making processes auditable.
Challenges and Future Directions
Despite its benefits, implementing Explainable AI is not without challenges. One significant hurdle is balancing the complexity of AI models with the need for transparency. Advanced models like deep learning neural networks are often highly accurate but difficult to interpret. Simplifying these models to enhance explainability may compromise their performance.
Another challenge lies in developing standardized methods for explainability across diverse applications. What works for healthcare AI may not be applicable to finance or defense. Tailored approaches are needed to address the unique requirements of each domain while maintaining consistency in transparency standards.
To address these challenges, ongoing research focuses on creating interpretable models that maintain high performance while providing clear explanations. Techniques such as LIME (Local Interpretable Model-agnostic Explanations) and SHAP (SHapley Additive exPlanations) are being widely adopted to improve model transparency. Additionally, collaboration between AI developers, domain experts, and policymakers is essential to establish guidelines and frameworks that promote explainability without sacrificing efficacy.
The future of XAI will likely involve the integration of user-friendly tools that enable non-technical stakeholders to interact with and understand AI systems. Visualizations, natural language explanations, and interactive dashboards are just a few examples of how XAI can be made more accessible to a broader audience.
Empowering Innovation: AI Solutions Beyond Gaming
At Ankatmak.ai, we specialize in IT consultancy and AI-driven solutions for all sectors. As a division of GameCloud Technologies, our focus is on leveraging artificial intelligence, software development, and prompt engineering to deliver custom innovations. With a commitment to results, we offer free pilot projects and a client-focused, success-driven approach. Our expertise ensures impactful, tailor-made solutions to address complex challenges across various industries.
Conclusion
Explainable AI plays a pivotal role in building trust in mission-critical decisions by making AI systems’ operations transparent and understandable. In sectors like healthcare, finance, and defense, where the consequences of AI decisions are profound, explainability is essential for fostering trust, ensuring fairness, and maintaining ethical standards.
As AI continues to integrate into critical areas, prioritizing explainability will be crucial to ensure that these systems are not only effective but also trustworthy and aligned with human values. By overcoming current challenges and advancing XAI technologies, we can pave the way for a future where AI serves as a reliable partner in making life-changing decisions.
For Know More Contact-Now
FAQ: What Role Do Explainable AI Models Play in Building Trust for Mission-Critical Decisions?
What is Explainable AI (XAI), and how does it differ from traditional AI models?
Explainable AI (XAI) refers to AI models designed to make their decision-making processes transparent and understandable to humans. Unlike traditional “black-box” models that provide outcomes without explanation, XAI clarifies how decisions are made, ensuring stakeholders can trust and verify the logic behind them.
Why is explainability important in mission-critical decisions?
In sectors like healthcare, finance, and defense, decisions driven by AI can have significant impacts on human lives and societal outcomes. Explainability builds trust by providing insights into the factors influencing AI decisions, ensuring they align with ethical standards, regulatory requirements, and user expectations.
What are the main challenges in implementing Explainable AI?
Key challenges include balancing model complexity and transparency, ensuring high performance while maintaining interpretability, and developing domain-specific explainability techniques. Additionally, creating standardized methods for diverse applications is an ongoing hurdle.
How does Explainable AI build trust among users and stakeholders?
XAI fosters trust by ensuring transparency, enabling accountability, and improving decision confidence. It allows stakeholders to verify AI outcomes, ensures ethical use, and provides clear explanations that facilitate collaboration and compliance with regulations.