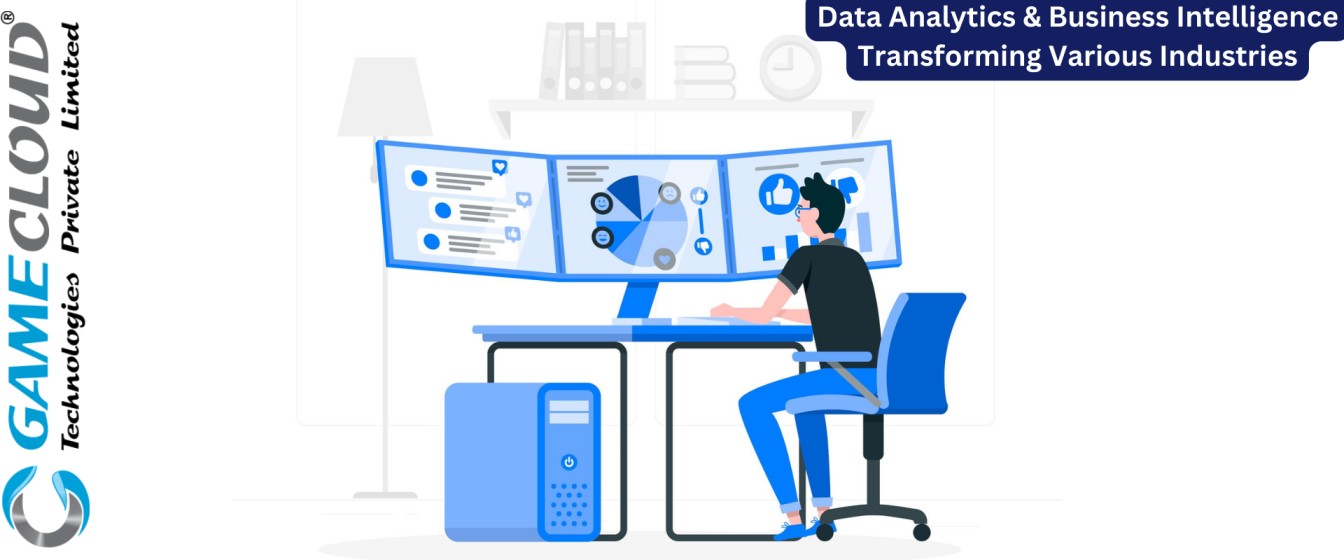
Data analytics and business intelligence have become crucial components of modern business operations, transforming the way organizations make decisions and operate. The integration of advanced technologies such as artificial intelligence (AI), machine learning (ML), and natural language processing (NLP) has significantly enhanced the capabilities of data analytics, enabling businesses to extract valuable insights from vast amounts of data. This blog aims to explore the transformative impact of data analytics and business intelligence on various industries, highlighting current trends, futuristic predictions, and real-world examples.
The Current Landscape of Data Analytics and Business Intelligence
The current landscape of data analytics and business intelligence is undergoing a transformative shift, driven by the rapid advancements in technology and the growing emphasis on data-driven decision-making. As organizations strive to stay competitive in an increasingly data-centric business environment, the adoption of cutting-edge tools and techniques in data analytics and business intelligence has become a strategic imperative.
According to a recent report by Knowledge Nile, the global business intelligence market is poised for significant growth in the coming years, with a projected compound annual growth rate (CAGR) of 11.4% from 2023 to 2028. This surge in market growth can be attributed to several key factors:
- The exponential increase in data generation: With the proliferation of digital technologies, the volume of data being generated by organizations has skyrocketed. From customer interactions to operational data, businesses are inundated with vast amounts of information that hold the potential to unlock valuable insights.
- The need for real-time decision-making: In today’s fast-paced business environment, organizations require the ability to make decisions quickly and effectively. Data analytics and business intelligence tools enable real-time data processing and analysis, allowing decision-makers to respond to market changes and customer demands in a timely manner.
- The rise of cloud-based solutions: The adoption of cloud-based data analytics and business intelligence platforms has gained significant traction in recent years. These solutions offer scalability, flexibility, and accessibility, making it easier for organizations to deploy and manage their data analytics infrastructure.
- The increasing focus on data governance and security: As organizations handle sensitive data, there is a growing emphasis on data governance and security measures. Data analytics and business intelligence tools are incorporating advanced security features and compliance standards to ensure the protection of critical data assets.
- The democratization of data: The traditional model of data analytics, where insights were primarily generated by specialized teams, is evolving. Data analytics and business intelligence tools are becoming more user-friendly and accessible, empowering employees across various departments to engage with data and make informed decisions.
- The integration of artificial intelligence and machine learning: The incorporation of AI and ML algorithms into data analytics and business intelligence platforms is revolutionizing the way insights are generated. These technologies enable advanced predictive modeling, anomaly detection, and natural language processing, allowing organizations to uncover hidden patterns and make more accurate forecasts.
- The emphasis on data visualization: Data visualization tools have become an integral part of the data analytics and business intelligence landscape. These tools enable the creation of intuitive, interactive dashboards and reports that facilitate better understanding and communication of data insights.
As the business intelligence market continues to grow, organizations are increasingly recognizing the strategic value of data analytics in driving innovation, improving operational efficiency, and enhancing customer experiences. By embracing the current trends and technologies in data analytics and business intelligence, businesses can position themselves for success in the rapidly evolving digital landscape.
Predictive Analysis for Data driven decision: Challenges and Impacts
When implementing predictive analytics, organizations may face a multitude of challenges that can hinder the successful integration of this powerful tool into their decision-making processes. One of the primary challenges is the sheer volume and complexity of data that needs to be collected, processed, and analyzed. With the exponential growth of data, organizations may struggle to identify the most relevant and valuable data points, leading to a lack of confidence in the accuracy of their predictions. Furthermore, the complexity of data can also lead to issues with data quality, as incorrect or incomplete data can significantly impact the reliability of predictive models.
Another significant challenge is the need for specialized skills and expertise. Predictive analytics requires a deep understanding of statistical modeling, data mining, and machine learning, which can be a significant barrier for organizations that lack the necessary talent or resources. This can lead to a reliance on external consultants or vendors, which can be costly and may not provide the level of customization and integration that organizations require. Additionally, the lack of internal expertise can also lead to a lack of understanding of the predictive models and their limitations, making it difficult for organizations to effectively communicate the results to stakeholders and make informed decisions.
The cost of implementing predictive analytics is another significant challenge. The software and hardware required to support predictive analytics can be expensive, and the need for high-performance computing and storage can be a significant burden on an organization’s IT infrastructure. Furthermore, the cost of training and maintaining the necessary skills and expertise can also be a significant challenge, particularly for organizations with limited budgets. The cost of implementing predictive analytics can also be a barrier to entry for smaller organizations or those with limited resources, making it difficult for them to compete with larger organizations that have the necessary resources to invest in this technology.
The lack of trust in predictive analytics is another significant challenge. Predictive analytics is a relatively new field, and many organizations may be hesitant to rely on models that are not yet fully understood. The lack of transparency and explainability of predictive models can also lead to a lack of trust, as organizations may not fully understand how the models are making predictions or how they can be improved. Additionally, the potential for bias in predictive models can also lead to a lack of trust, particularly in organizations that are subject to regulatory requirements or have a high level of public scrutiny.
The need for ongoing maintenance and updates is another significant challenge. Predictive models are not static and require ongoing maintenance and updates to ensure that they remain accurate and relevant. This can be a significant challenge for organizations that lack the necessary resources or expertise to maintain and update their predictive models. The need for ongoing maintenance and updates can also lead to a lack of confidence in the accuracy of predictive models, as organizations may not have the necessary resources to ensure that the models remain up-to-date and accurate.
The lack of integration with existing systems and processes is another significant challenge. Predictive analytics is often a standalone solution that may not integrate seamlessly with an organization’s existing systems and processes. This can lead to a lack of consistency and coordination between predictive analytics and other business functions, making it difficult for organizations to fully leverage the benefits of predictive analytics. The lack of integration can also lead to a lack of trust in predictive analytics, as organizations may not fully understand how the models are being used or how they can be integrated with other business functions.
The need for a cultural shift is another significant challenge. Predictive analytics requires a cultural shift within an organization, as it requires a willingness to rely on data-driven insights rather than intuition or experience. This can be a significant challenge for organizations that are used to making decisions based on gut feelings or experience, as it requires a significant change in mindset and approach. The need for a cultural shift can also lead to resistance to change, as some employees may be hesitant to adopt new approaches and technologies.
Here are some ways predictive analytics can be used to make sound data-driven decisions:
- Risk Reduction: Predictive analytics can help identify potential risks and uncertainties in advance. This proactive approach enables organizations to implement preventive measures and safeguards, reducing the likelihood of negative outcomes.
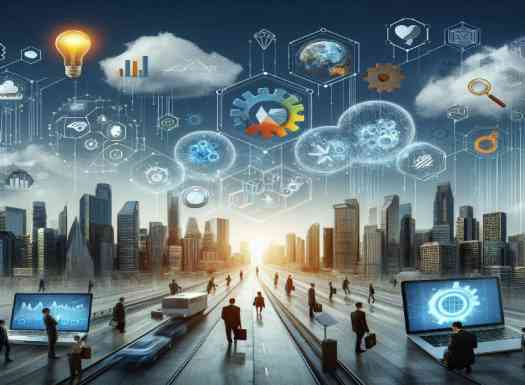
- Improved Forecasting: Predictive analytics can be used to forecast future trends, market conditions, and customer behaviors. This foresight enables proactive decision-making, allowing businesses to adapt to changing circumstances and stay ahead of the competition.
- Optimized Resource Allocation: By understanding future trends and demands, predictive analytics helps in optimizing resource allocation. This ensures that resources are allocated efficiently and effectively, leading to cost savings and improved operational efficiency.
- Personalized Decision Support: Predictive analytics enables personalized decision support by analyzing individual customer behaviors and preferences. This level of customization allows businesses to tailor their strategies, marketing efforts, and product offerings to meet the specific needs of their target audience.
- Enhanced Accuracy and Precision: Predictive analytics leverages advanced algorithms to analyze vast amounts of data, providing more accurate and precise insights. Decision-makers can rely on data-driven predictions rather than gut feelings, reducing the risk of errors in judgment.
- Strategic Planning and Competitive Advantage: Integrating predictive analytics into decision-making processes empowers organizations with strategic insights. Businesses can align their strategies with market trends, customer expectations, and competitor movements, gaining a competitive advantage in the dynamic business landscape.
- Transformative Impact on Business, Decision-Making, and Society: Predictive analytics is not merely a technological innovation; it stands as a transformative force reshaping the landscape of business, decision-making, and society.
- Continuous Improvement: The iterative nature of predictive analytics allows for continuous improvement. As more data becomes available, models can be refined, leading to increasingly accurate predictions and better-informed decision-making over time.
- Data-Driven Decision-Making: Predictive analytics empowers organizations to base decisions on data insights rather than intuition, improving accuracy and strategic planning.
- Proactive Approach: Predictive analytics enables businesses to adopt a proactive approach by anticipating future events, enabling them to take preemptive actions.
By incorporating predictive analytics into the decision-making process, organizations can make more informed, data-driven decisions that drive business success and stay ahead of the competition.
Industry-Specific Applications of Data Analytics and Business Intelligence
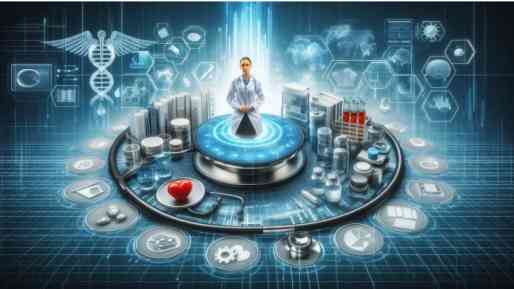
- Healthcare
In the healthcare sector, data analytics and business intelligence play a pivotal role in enhancing patient care, operational efficiency, and cost management. Beyond the mentioned benefits, data analytics is revolutionizing healthcare by enabling predictive modeling for disease outbreaks, personalized treatment plans, and resource optimization. For instance, predictive analytics can forecast patient admission rates, allowing hospitals to allocate resources effectively and improve patient flow. Moreover, data-driven insights help in identifying high-risk patients for proactive interventions, reducing readmission rates and improving overall healthcare outcomes.
- Finance
Data analytics and business intelligence have transformed the financial industry by enabling more informed decision-making, risk management, and strategic investments. In addition to the highlighted advantages, these technologies are instrumental in fraud detection, algorithmic trading, and customer segmentation. For example, predictive analytics can identify fraudulent activities by analyzing transaction patterns, enhancing security measures and safeguarding financial institutions. Moreover, machine learning algorithms can optimize investment portfolios by predicting market trends and identifying profitable opportunities, leading to improved financial performance and risk mitigation.
- Retail
The retail sector leverages data analytics and business intelligence to drive customer engagement, streamline operations, and boost profitability. In addition to the mentioned benefits, these technologies are reshaping retail through dynamic pricing strategies, demand forecasting, and personalized marketing campaigns. For instance, predictive analytics can analyze customer behavior to tailor product recommendations, enhancing the shopping experience and increasing customer loyalty. Furthermore, data-driven insights enable retailers to optimize inventory levels, reduce stockouts, and improve supply chain efficiency, ultimately leading to enhanced sales performance and cost savings.
- Manufacturing
In the manufacturing industry, data analytics and business intelligence are revolutionizing production processes, supply chain management, and maintenance operations. Beyond the highlighted advantages, these technologies enable predictive maintenance, quality control, and demand forecasting. For example, predictive analytics can analyze equipment sensor data to predict maintenance needs, reducing downtime and optimizing production efficiency. Moreover, data-driven insights help manufacturers identify production bottlenecks, streamline workflows, and improve product quality, leading to increased operational efficiency and cost savings.
- Telecommunications
Data analytics and business intelligence are driving innovation in the telecommunications sector by enhancing network performance, customer service, and marketing strategies. In addition to the mentioned benefits, these technologies are instrumental in network optimization, customer churn prediction, and targeted marketing campaigns. For example, predictive analytics can analyze network data to predict potential failures, enabling proactive maintenance and minimizing service disruptions. Moreover, data-driven insights help telecommunications companies personalize customer interactions, improve service offerings, and optimize marketing efforts, ultimately leading to enhanced customer satisfaction and retention.
In each of these industries, the strategic application of data analytics and business intelligence is reshaping traditional practices, driving efficiency, and fostering innovation to stay competitive in today’s data-driven business landscape.
Data Analytics and Business Intelligence in Supply Chain Management: A Special Case Study
In the supply chain management industry, leveraging data analytics and business intelligence offers a multitude of benefits that drive operational efficiency, enhance decision-making, and improve overall performance. Here are some key advantages:
- Improved Decision-Making: Data analytics and business intelligence provide valuable insights that empower decision-makers to make informed choices. By analyzing data from multiple sources, organizations can delve into customer behaviors, business performance, and industry trends, enabling them to make strategic decisions that drive efficiency and profitability.
- Enhanced Operational Efficiency: Business intelligence tools offer a comprehensive view of all operations, allowing companies to identify bottlenecks, streamline processes, and optimize performance. By gaining visibility into the entire supply chain, organizations can identify inefficiencies, reduce costs, and improve overall operational efficiency.
- Predictive Analytics for Forecasting: Predictive analytics tools enable organizations to anticipate market changes, demand fluctuations, and supply chain disruptions. By leveraging predictive modeling, businesses can proactively address potential issues, optimize inventory levels, and improve supply chain resilience.
- Real-Time Reporting and Monitoring: Data analytics and business intelligence tools provide real-time insights into supply chain operations, allowing stakeholders to monitor performance, track progress, and make timely adjustments. This real-time visibility enables organizations to respond quickly to changing market conditions and customer demands.
- Cost Optimization and Revenue Maximization: By using targeted strategies derived from data analytics, organizations can identify new revenue opportunities, optimize pricing strategies, and maximize profitability. Data-driven decision-making helps in cost optimization, resource allocation, and revenue generation, leading to improved financial performance.
- Risk Mitigation: Analyzing trends and historical data enables organizations to better understand risks and potential threats in the supply chain. By identifying vulnerabilities and anticipating disruptions, businesses can implement proactive measures to mitigate risks, enhance resilience, and ensure continuity of operations.
- Customer Insights and Satisfaction: Business intelligence tools help organizations gain essential customer insights, understand customer preferences, behaviors, and needs. By analyzing customer data, organizations can tailor their products, services, and marketing strategies to meet customer expectations, drive customer satisfaction, and enhance brand loyalty.
- Supply Chain Transparency: Data analytics and business intelligence promote transparency in the supply chain, allowing organizations to visualize the flow of goods, monitor supplier performance, and identify potential concerns before they escalate into problems. This transparency fosters trust among stakeholders and enables effective collaboration across the supply chain network.
The strategic utilization of data analytics and business intelligence in supply chain management empowers organizations to optimize operations, drive innovation, and adapt to dynamic market conditions, ultimately leading to improved efficiency, profitability, and competitiveness in the industry.
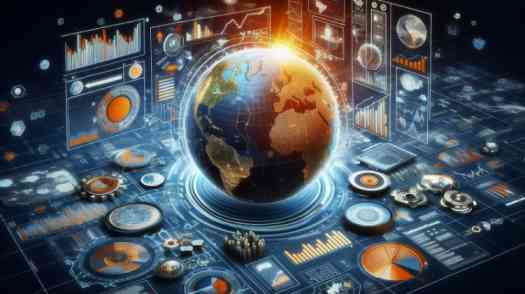
Current Trends and Predictions in Data Analytics and Business Intelligence
- Augmented Analytics
Augmented analytics is a rapidly growing trend that leverages artificial intelligence (AI) and machine learning (ML) to automate various aspects of the data analytics process. This approach aims to enhance human intelligence by automating data preparation, insight discovery, and reporting.
According to a recent report by Knowledge Nile, the global augmented analytics market is expected to grow at a compound annual growth rate (CAGR) of 30.4% from 2023 to 2028. This significant growth can be attributed to the increasing demand for data-driven decision-making and the need for more efficient and effective analytics processes.
Augmented analytics enables businesses to gain insights more quickly and accurately by automating time-consuming tasks such as data cleaning, feature engineering, and model selection. By leveraging AI and ML algorithms, augmented analytics can identify patterns and trends in large datasets that may be difficult for humans to detect.
Moreover, augmented analytics can generate natural language explanations of insights, making it easier for non-technical users to understand and act upon the findings. This democratization of data analytics empowers employees across various departments to make data-driven decisions, leading to improved business outcomes.
- Real-Time Analytics
Real-time analytics is another significant trend in data analytics and business intelligence. This approach involves the use of advanced technologies such as the Internet of Things (IoT) and cloud computing to analyze large amounts of data in real-time.
According to a recent report by , the global real-time analytics market is expected to grow at a CAGR of 24.5% from 2023 to 2028. This growth is driven by the increasing need for organizations to make decisions quickly in response to rapidly changing market conditions and customer demands.
Real-time analytics enables businesses to monitor and analyze data streams from various sources, such as IoT devices, social media, and customer interactions. By processing and analyzing this data in real-time, organizations can gain immediate insights and take action to address emerging issues or capitalize on new opportunities.
For example, in the retail industry, real-time analytics can help businesses optimize pricing strategies based on real-time demand data, leading to increased revenue and customer satisfaction. In the manufacturing sector, real-time analytics can be used to monitor equipment performance and predict maintenance needs, reducing downtime and improving efficiency.
- Data Democratization
Data democratization is a key prediction in the field of data analytics and business intelligence. This trend involves the widespread adoption of self-service analytics tools and the empowerment of non-technical users to perform data analysis.
According to that same report , the global data democratization market is expected to grow at a CAGR of 25.6% from 2023 to 2028. This growth is driven by the increasing need for organizations to make data-driven decisions at all levels of the organization, not just at the executive level.
Data democratization enables employees across various departments to access, analyze, and visualize data without relying on specialized IT or data science teams. By providing user-friendly self-service analytics tools, organizations can empower their employees to make data-driven decisions more quickly and effectively.
Moreover, data democratization can lead to improved collaboration and communication across the organization, as employees from different departments can share insights and work together to solve problems. This can lead to improved decision-making, increased innovation, and better business outcomes.
- Predictive and Prescriptive Analytics
Predictive and prescriptive analytics are two closely related trends that are gaining traction in the field of data analytics and business intelligence. Predictive analytics involves the use of statistical models and machine learning algorithms to forecast future events or outcomes based on historical data.
Prescriptive analytics builds upon predictive analytics by providing recommendations on the best course of action to achieve a desired outcome. By analyzing vast amounts of data and considering multiple scenarios, prescriptive analytics can help organizations make more informed decisions and optimize their strategies.
Predictive and prescriptive analytics have applications across various industries, from finance to healthcare to retail. In the finance sector, these approaches can be used to assess credit risk, detect fraud, and optimize investment strategies. In healthcare, predictive analytics can help identify high-risk patients and tailor preventive care strategies, while prescriptive analytics can guide treatment decisions.
- Edge Computing and Internet of Things (IoT)
Edge computing and the Internet of Things (IoT) are two closely related trends that are transforming the way data is collected, processed, and analyzed. Edge computing involves processing data closer to the source, rather than in a centralized data center or cloud. This approach reduces latency, improves response times, and enables real-time decision-making.
IoT refers to the network of connected devices that collect and transmit data from various sources, such as sensors, cameras, and wearables. By combining edge computing and IoT, organizations can process and analyze data in real-time, closer to the point of data generation. This can lead to improved efficiency, reduced costs, and enhanced decision-making.
Edge computing and IoT have applications across various industries, from manufacturing to healthcare to smart cities. In manufacturing, IoT sensors can monitor equipment performance and trigger maintenance alerts, while edge computing can enable real-time quality control and process optimization. In healthcare, wearable devices can monitor patient health and trigger alerts to caregivers, while edge computing can enable real-time analysis of patient data and personalized treatment recommendations.
- Data Governance and Security
As organizations collect and store more data than ever before, the importance of data governance and security is becoming increasingly critical. Data governance involves establishing policies, standards, and processes for managing data throughout its lifecycle, from creation to storage to disposal.
Data security is essential for protecting sensitive data from unauthorized access, theft, or misuse. With the increasing threat of cyber attacks and data breaches, organizations must implement robust security measures such as encryption, access controls, and threat detection.
Data governance and security are not only important for compliance and risk management but also for building trust with customers and stakeholders. By demonstrating a commitment to data privacy and security, organizations can enhance their reputation and competitive advantage in the market.
The field of data analytics and business intelligence is rapidly evolving, driven by the increasing adoption of advanced technologies such as AI, ML, IoT, and edge computing. As organizations continue to generate and collect vast amounts of data, the need for more efficient, effective, and secure analytics solutions will only grow. By embracing these current trends and predictions, organizations can gain a competitive edge, drive innovation, and achieve better business outcomes.
Real-World Examples of Business Intelligence and Analytics Implementation
- Coca-Cola: Coca-Cola is a prime example of a company that has effectively utilized business intelligence and analytics to enhance its operations and drive growth. The multinational beverage company leverages BI tools to gather and analyze vast amounts of data, enabling them to improve marketing strategies, streamline operations, and enhance customer engagement.
One notable initiative by Coca-Cola is the “Coke On” loyalty program in Japan, where they analyze purchase data to offer personalized rewards, boosting customer retention and loyalty. Additionally, the company uses BI tools for demand forecasting based on historical sales data, allowing them to predict trends and optimize production schedules for increased operational efficiency.
Moreover, Coca-Cola’s “Share a Coke” campaign showcases how BI and analytics were used to personalize marketing efforts by printing consumer names on bottles, tracking popular names, and adjusting production accordingly to drive customer engagement and brand loyalty.
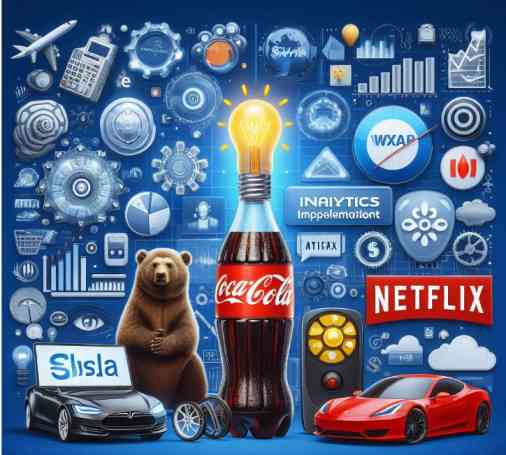
- Walmart: Walmart is a leading example of a company that has harnessed the power of business intelligence to optimize operations and drive business success. With a vast amount of customer data from its stores and websites, Walmart implemented BI tools to centralize and analyze this data for actionable insights.
Walmart’s transition to a single website and a 250-node Hadoop cluster for online customer data storage demonstrates how BI helped unify data sources for better analysis and decision-making. By streamlining and visualizing data, managers and executives could understand the numbers and make effective decisions to influence key business outcomes.
BI tools at Walmart facilitated product placement strategies, competitive pricing decisions, and real-time monitoring of market trends. By leveraging BI for data-driven decision-making, Walmart gained a competitive advantage in the retail industry and improved customer experiences through targeted campaigns and optimized operations.
- Netflix: Netflix is a standout example of a company that has revolutionized its industry through the strategic use of data analytics and business intelligence. The streaming service giant leverages BI tools to personalize content recommendations, optimize content creation, and enhance customer retention.
Netflix’s recommendation engine is a key component of its data-driven strategy, analyzing viewer data to provide personalized content suggestions that drive customer engagement and satisfaction. By tracking viewer behavior and preferences, Netflix tailors its content offerings to individual tastes, increasing viewer retention and loyalty.
Furthermore, Netflix uses BI for content creation strategies, analyzing viewer data to identify trends, predict demand, and create content that resonates with its audience. This data-driven approach has led to the creation of hit shows that drive subscriber growth and reinforce Netflix’s position as a leader in the streaming industry.
- Tesla: Tesla exemplifies how data analytics and business intelligence can drive innovation and enhance customer experiences in the automotive industry. The electric vehicle manufacturer utilizes advanced analytics to monitor vehicle performance, optimize maintenance, and personalize customer interactions.
Tesla’s vehicles are equipped with sensors that collect real-time data on performance and driver behavior, enabling the company to analyze this data for insights on vehicle optimization and future product development. By leveraging BI tools, Tesla gains a comprehensive understanding of vehicle performance and customer preferences, leading to improved product offerings and customer satisfaction.
Moreover, Tesla uses data analytics to personalize customer experiences, offering tailored software updates, maintenance recommendations, and customer support based on individual preferences and feedback. This data-driven approach enhances the overall ownership experience and strengthens customer loyalty.
These real-world examples showcase the transformative impact of business intelligence and analytics across various industries, highlighting how data-driven strategies can optimize operations, drive growth, and enhance customer engagement.
Data Analytics and Business Intelligence in Gaming Industry
Data analytics and business intelligence are transforming the gaming industry by providing valuable insights into player behavior, preferences, and engagement. Here are some key ways gaming companies are leveraging data analytics and BI:
- Player Sentiment Analysis: One of the most significant applications of AI in gaming is player sentiment analysis, which involves analyzing player feedback, reviews, and other data to gain valuable insights into their preferences for game levels, menu elements, or opponents. By harnessing the capabilities of AI sentiment analysis, game developers can scrutinize player feedback to discern what aspects of games resonate most with them and what needs to be refined.
- Procedural Content Generation (PCG): AI can revolutionize the process of generating game assets like 3D objects, characters, levels, and quests. By using AI in PCG, game developers can craft richer, more diverse worlds, simplifying the complex process of game asset generation at an accelerated rate to meet users’ demands. AI can also generate interactive narratives based on past storylines, as seen in the text-based adventure game AI Dungeon 2.
- Player-Experience Modeling (PEM): PEM mathematically models gamers’ experience and anticipates their preferences. AI in player experience modeling analyzes users’ competence and emotional status to adjust the gaming mechanism accordingly in real-time. As per the gamers’ skill level, AI can increase or decrease the game’s complexity, making it more interactive and adaptive to users’ interests.
- Data Mining and Real-Time Analytics: The vast amounts of data generated by millions of gamers globally can be harnessed using AI and machine learning for data mining and real-time analytics. By deploying AI to analyze real-time gaming data, companies can extract actionable insights to optimize the gaming experience, address player concerns, and seize opportunities in a timely manner.
- Cheat Detection in Multiplayer Games: Cheating has been a significant challenge in multiplayer games, negatively impacting the player experience. AI can analyze player movement patterns and detect unfair tactics, ensuring a secure and fair gaming environment. Games like PUBG already use AI to prevent cheating and maintain the integrity of the gameplay.
By leveraging data analytics and business intelligence, gaming companies can gain a deeper understanding of player preferences, optimize game design, enhance player engagement, and stay ahead of the competition. As AI continues to advance, we can expect to see even more innovative applications of data-driven insights in the gaming industry.
Ankatmak: The Pioneer in Industry Transformation
Ankatmak, a division of GameCloud Technologies Pvt Ltd, stands at the forefront of leveraging data analytics and business intelligence to drive transformative changes across industries. Specializing in AI outsourcing, prompt engineering, and IT consultancy, Ankatmak offers a diverse range of services, including software development, website development, custom software solutions, IT consulting, cloud services, and data analytics. With a team of over 70 dedicated professionals equipped with research, design, and development skills, Ankatmak embodies GameCloud’s vision of harnessing AI for innovative solutions across various technical domains. By providing tailored, cutting-edge AI and IT consultancy services, Ankatmak is at the forefront of utilizing data analytics and business intelligence to revolutionize industries and drive sustainable growth.
Conclusion
In conclusion, the dynamic landscape of business intelligence and data analytics is reshaping industries worldwide, driving innovation, efficiency, and strategic decision-making. As organizations harness the power of advanced technologies like AI, machine learning, and real-time analytics, they are unlocking new opportunities for growth, sustainability, and competitive advantage. The transformative impact of data-driven insights is evident across sectors such as healthcare, finance, retail, and beyond, where personalized experiences, optimized operations, and predictive strategies are becoming the norm. Embracing this data-driven revolution is not just a choice but a necessity for organizations looking to thrive in the digital age. By continuously evolving their data analytics capabilities and leveraging business intelligence effectively, companies can navigate complexities, seize opportunities, and lead the way in transforming industries for a brighter, data-driven future.