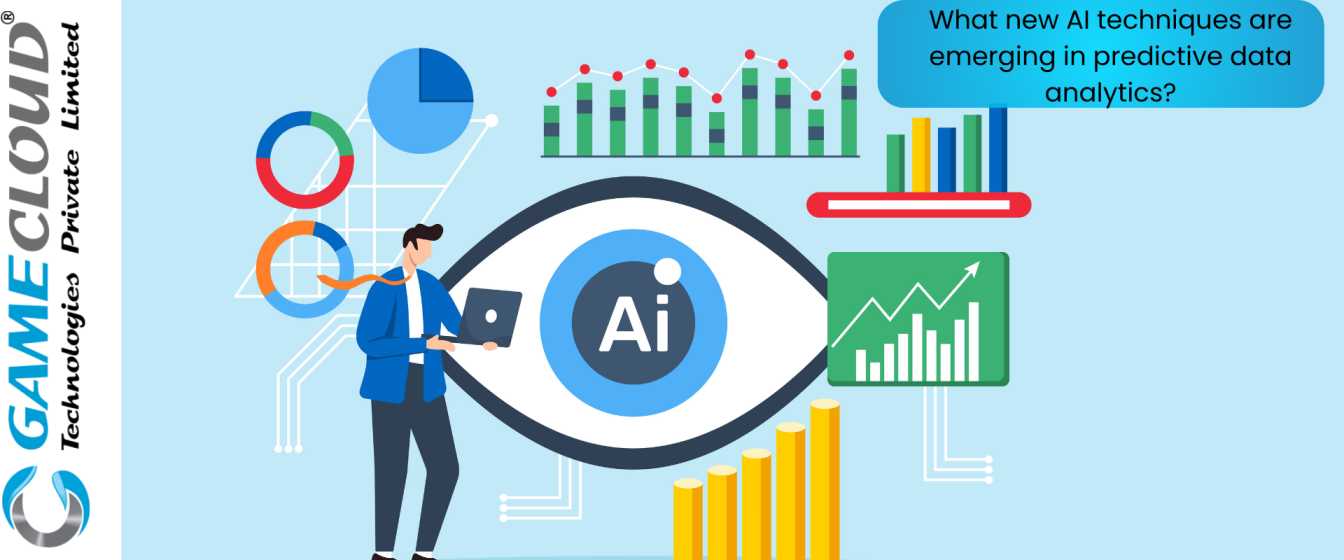
Predictive data analytics is a crucial tool for organizations, enabling them to anticipate future trends by analyzing historical data. It is widely used across industries such as healthcare, finance, and marketing, helping improve decision-making and operational efficiency.
AI has significantly transformed predictive analytics by processing vast datasets and detecting intricate patterns that traditional methods may miss. For instance, AI algorithms can analyze social media trends, customer behavior, and market changes in real time, resulting in more accurate forecasts. Moreover, AI models continuously learn and adapt as new data is introduced, improving prediction accuracy over time. These advancements help reduce forecasting errors and save time and costs for businesses.
With AI’s capability to automate tasks and identify hidden trends, predictive analytics is becoming more reliable and impactful, offering companies a competitive advantage in today’s data-driven world.
Deep Learning and Neural Networks in Predictive Analytics
Deep learning and neural networks are transforming predictive data analytics by mimicking the brain’s ability to process vast amounts of information. Convolutional Neural Networks excel in recognizing patterns within images and are widely used in healthcare for diagnosing diseases from medical scans. In finance, Recurrent Neural Networks (RNNs) and Long Short-Term Memory (LSTM) networks process sequential data, which is crucial for predicting stock market trends and financial forecasting. Generative Adversarial Networks (GANs) also play a significant role by creating realistic data simulations, enhancing model accuracy in industries like retail for customer behavior forecasting.
Reinforcement Learning and Transfer Learning for Enhanced Predictions
Reinforcement Learning and Transfer Learning are transforming predictive data analytics by offering more adaptive and efficient prediction models. RL excels in dynamic environments by using trial-and-error learning, where a system optimizes decisions based on rewards and penalties. This approach is particularly valuable in industries where conditions are constantly changing. For instance, RL can adjust inventory levels or trading strategies in real time, maximizing efficiency and profitability.
Transfer Learning, on the other hand, allows AI models to leverage pre-trained knowledge from one domain and apply it to another. This reduces the need for large, domain-specific datasets, which is a major advantage in fields like healthcare or finance, where labeled data can be scarce. By transferring learned patterns from similar domains, Transfer Learning improves model accuracy and speeds up deployment, enhancing the efficiency of predictive systems. Both techniques are paving the way for more robust and scalable predictions.
Innovative AI Solutions: Tailored for Your Business Success
At Ankatmak, the AI & IT arm of GameCloud, we provide cutting-edge AI-driven content creation, IT consultancy, and custom software solutions to help businesses excel in the digital world. Whether it’s chatbot development, data analytics, or AI outsourcing, our expert team ensures you only pay when you’re fully satisfied. Backed by over 70 skilled professionals, we are dedicated to delivering innovative, tailored solutions that meet your unique business needs, keeping you ahead in today’s competitive landscape.
Conclusion
In conclusion, AI techniques such as deep learning, reinforcement learning, and transfer learning are revolutionizing predictive data analytics by improving accuracy and adaptability across industries. However, challenges like data privacy and algorithmic bias must be addressed. As AI continues to evolve, it promises to further enhance predictive analytics, making it more ethical, efficient, and scalable.
For Know More Contact-Now
FAQ: What new AI techniques are emerging in predictive data analytics?
1. What is predictive data analytics and why is it important?
Predictive data analytics involves analyzing historical data to forecast future trends and behaviors. It is essential for organizations as it enhances decision-making, improves operational efficiency, and provides insights that help in anticipating market changes, customer needs, and potential challenges across various industries such as healthcare, finance, and marketing.
2. How has AI transformed predictive analytics?
AI has revolutionized predictive analytics by processing large datasets and identifying complex patterns that traditional methods often overlook. AI algorithms can analyze real-time data from various sources, such as social media and customer interactions, leading to more accurate forecasts. Additionally, AI models continuously learn from new data, improving their prediction accuracy over time.
3. What are deep learning and neural networks, and how do they impact predictive analytics?
Deep learning and neural networks are advanced AI techniques that simulate human brain functions to process vast amounts of information. Convolutional Neural Networks (CNNs) are effective in image pattern recognition, crucial for medical diagnoses, while Recurrent Neural Networks (RNNs) and Long Short-Term Memory (LSTM) networks excel in sequential data analysis, making them suitable for stock market predictions. These technologies enhance the accuracy of predictive models across various industries.
4. What are reinforcement learning and transfer learning, and how do they improve predictions?
Reinforcement Learning (RL) uses a trial-and-error approach to optimize decision-making in dynamic environments, making it valuable for industries with constantly changing conditions. For example, it can help adjust inventory levels in real time. Transfer Learning allows AI models to apply knowledge gained from one domain to another, reducing the need for large, specific datasets and speeding up model deployment. Both techniques contribute to more robust and efficient predictive systems.
5. What challenges does AI in predictive analytics face, and what is the future outlook?
While AI techniques significantly improve predictive analytics, challenges like data privacy concerns and algorithmic bias must be addressed to ensure ethical use. The future of AI in predictive analytics looks promising, with advancements expected to enhance model accuracy, adaptability, and scalability, making predictive analytics more effective and ethical in various applications.