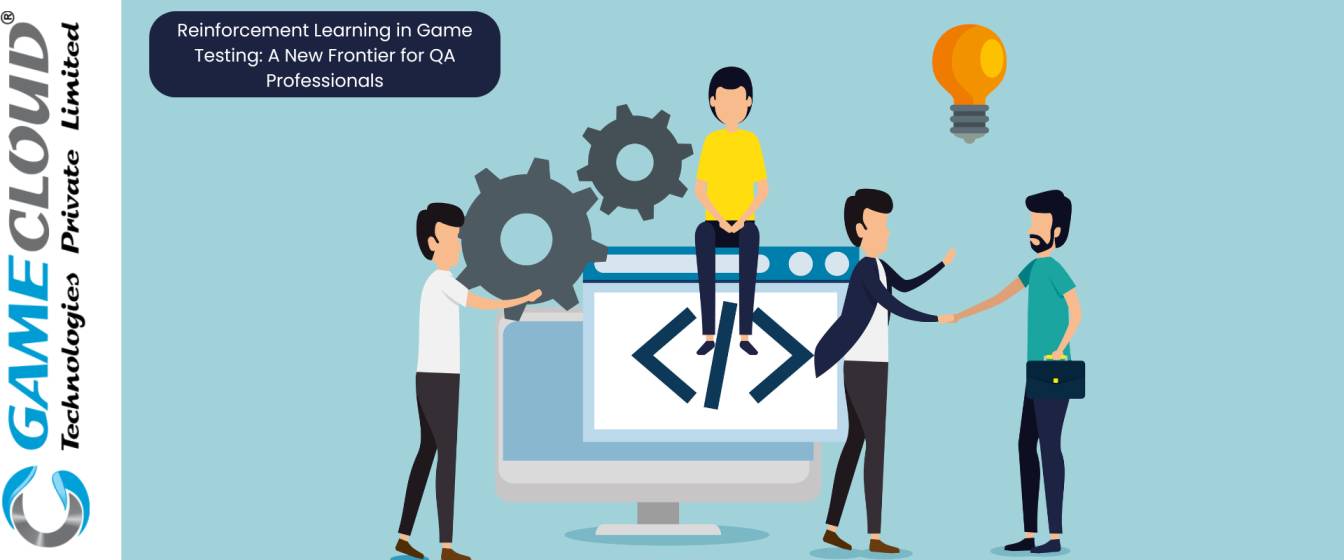
The field of QA in gaming has seen significant advancements, and one of the most exciting developments is the use of Reinforcement Learning. By leveraging this branch of AI, QA teams can now test games in ways that were previously impossible. RL allows machines to learn how to play and interact with games autonomously, which can significantly improve the testing process.
What Is Reinforcement Learning?
Reinforcement Learning is a machine learning technique where an agent learns by interacting with its environment. Through trial and error, the agent gains insights into what actions lead to positive outcomes and which actions yield negative results. Over time, it learns to make better decisions to achieve specific goals.
In the context of game testing, RL agents are programmed to interact with the game, mimicking human players. These agents don’t require predefined instructions—they explore environments dynamically, making them highly effective for finding issues beyond scripted scenarios.
How Reinforcement Learning Improves Game Testing
Reinforcement Learning is transforming game testing in multiple ways, offering new insights and automation capabilities.
1. Exploring Beyond Scripted Testing
Traditional game testing often involves manual efforts or prewritten automated scripts. These methods are limited in their ability to explore unexpected player actions or edge cases. RL agents, on the other hand, operate with no predefined boundaries, enabling them to uncover bugs and glitches that traditional methods might miss. For example, in a platformer game, an RL agent might find areas where characters can unintentionally bypass obstacles—potential exploits that QA testers could overlook.
2. Uncovering Game Exploits
Exploits—ways players bend game mechanics to gain unfair advantages—can harm gameplay balance and lead to dissatisfaction among players. RL agents can simulate a wide variety of player behaviors to test mechanics for vulnerabilities. By identifying exploits during the testing phase, developers can address these issues before the game reaches the market.
3. Optimizing Game Balance
Balancing a game is an art as much as it is a science, and achieving the right balance is critical for player satisfaction.Game balance is critical to delivering a satisfying experience. RL agents can simulate countless playthroughs, revealing how certain mechanics might give an unfair advantage or how difficulty might fluctuate across levels. This data helps developers make necessary adjustments, creating a more enjoyable experience for players.
For instance, RL can evaluate the difficulty of puzzles in an adventure game and identify areas that might feel too frustrating or too easy for players, providing actionable insights for improvement.
4. Automation for Regression Testing
Automating repetitive testing tasks is one of the strongest advantages RL brings to game QA. When a game is updated or patched, regression testing ensures the new changes haven’t broken any existing features. RL agents can automate this process by re-playing affected sections of the game. This not only speeds up the process but also allows human testers to focus on more complex aspects of QA, such as storyline cohesion or the emotional impact of a game.
The Challenges of Using Reinforcement Learning in QA
While the benefits of RL are significant, its implementation in game testing isn’t without obstacles:
- High Computational Requirements: Training RL agents requires significant processing power and time. This can be costly for smaller teams or indie developers.
- Realism in Player Simulation: RL agents, while powerful, may not perfectly replicate human behavior. Ensuring they act in ways that reflect real player interactions is essential for effective testing.
- Specialized Knowledge: Integrating RL into a QA process demands expertise in machine learning, which many traditional QA teams may lack.
Despite these challenges, advancements in RL technologies are making its integration into game testing more accessible over time.
Future Possibilities in RL-Powered Game Testing
The future of reinforcement learning in game testing holds immense promise, with potential applications expanding rapidly. As RL continues to evolve, its potential applications in game testing are expanding. Future RL agents may be capable of:
- Simulating emotional responses to narrative elements, giving developers feedback on story pacing and player engagement.
- Exploring open-world games to evaluate dynamic environmental interactions.
- Testing multiplayer environments for balance and fairness in competitive play.
These advancements could take game testing to a whole new level, making games not only more polished but also more immersive.
Why QA Professionals Should Embrace Reinforcement Learning
Reinforcement Learning isn’t just a tool for finding bugs—it’s a method for understanding the entire gameplay experience. QA professionals who incorporate RL into their workflows can deliver higher-quality results in less time. This doesn’t mean human testers are being replaced. Instead, RL frees them from repetitive tasks, allowing them to focus on creative and subjective aspects of testing that machines can’t replicate.
Your Partner in Game Quality Assurance and Success
At GameCloud Technologies Pvt Ltd., we are dedicated to ensuring your game’s success at every stage. Since 2011, we’ve tested and validated over 1,000 games and apps across various platforms. Our team of certified QA professionals and passionate gamers provides comprehensive services, including functionality, compatibility, and performance testing. We offer fully equipped solutions without upfront payments or minimum purchase requirements. Trust us to safeguard your monetization strategy and deliver a seamless gaming experience to your users.
The Role of Automation and AI in Game Testing
Automation and AI have started to transform the game testing landscape. Automated testing tools can quickly identify common issues, speeding up the overall process. Meanwhile, AI-driven systems analyze game performance and player behavior, offering insights that traditional methods may miss.
Although human testers remain indispensable for assessing creative and subjective elements, the integration of these technologies is expected to make testing more efficient and cost-effective, further driving market growth.
Regional Market Growth
The game testing service market is seeing significant growth in regions like Asia-Pacific, Europe, and North America. Asia-Pacific, in particular, has emerged as a hotspot for gaming, with countries like China, Japan, and India boasting large gaming populations. This has spurred demand for localized testing services to cater to regional languages and cultural nuances.
Similarly, North America and Europe continue to be major players in the market, with high adoption rates of advanced gaming technologies and a strong focus on delivering top-tier gaming experiences.
Future Outlook
By 2031, the game testing service market is expected to witness steady growth, driven by the evolving demands of the gaming industry. As technology advances and games become more complex, the importance of professional testing services will only increase. With automation and AI becoming integral to the testing process, the market is poised for sustained expansion.
For game developers and publishers, investing in reliable testing services is no longer optional. It’s an essential step toward ensuring their games succeed in an increasingly competitive market.
GameCloud: Your Partner in Perfecting Video Games
At GameCloud, we specialize in delivering top-notch video game quality assurance. With over a decade of expertise and 1000+ games tested, our certified QA professionals ensure flawless gameplay, seamless functionality, and optimal performance across all platforms, including VR and consoles. From initial development to post-launch, we’re dedicated to safeguarding your game’s quality and enhancing user satisfaction. Trust GameCloud for competitive pricing, cutting-edge tools, and a passionate team committed to your game’s success.
Conclusion
Reinforcement Learning is reshaping the way QA professionals approach game testing. From identifying exploits to optimizing game balance and automating regression testing, RL brings a host of advantages to the table. While challenges exist, the future of RL in game testing is bright, offering the potential for smarter, more efficient QA processes. For developers and QA teams looking to stay ahead, exploring RL as a testing tool is an opportunity worth considering. It’s not just about keeping up with trends—it’s about delivering better, more engaging games for players worldwide.
For Know More Contact-Now
FAQs: Reinforcement Learning in Game Testing: A New Frontier for QA Professionals
How does Reinforcement Learning (RL) differ from traditional automated game testing?
Reinforcement Learning differs from traditional automated game testing by allowing AI agents to explore and interact with the game environment without relying on predefined scripts. Unlike scripted testing methods that follow specific scenarios, RL agents learn through trial and error, adapting dynamically to new situations. This makes RL highly effective in uncovering hidden bugs, exploits, and unexpected gameplay behaviors that scripted tests might miss.
Can Reinforcement Learning completely replace human QA testers?
No, Reinforcement Learning is not meant to replace human QA testers but rather to enhance their efficiency. RL automates repetitive tasks like regression testing and game balance assessments, allowing human testers to focus on subjective aspects such as storytelling, user experience, and creative gameplay evaluation. The combination of RL-powered automation and human expertise ensures a more thorough testing process.
What are the key challenges of implementing RL in game testing?
Implementing RL in game testing comes with several challenges, including high computational requirements, the need for specialized machine learning expertise, and ensuring that RL agents simulate realistic player behavior. Training RL models demands significant processing power and time, making it a costly investment, especially for smaller studios. However, as RL technology advances, these barriers are gradually being reduced.
How can RL improve game balance and fairness?
RL agents can simulate thousands of gameplay sessions to analyze how different mechanics affect game balance. By detecting overpowered abilities, unintended difficulty spikes, or unfair advantages, RL helps developers fine-tune the game for a more balanced and enjoyable experience. This is particularly useful in multiplayer games, where fairness is crucial for competitive integrity.